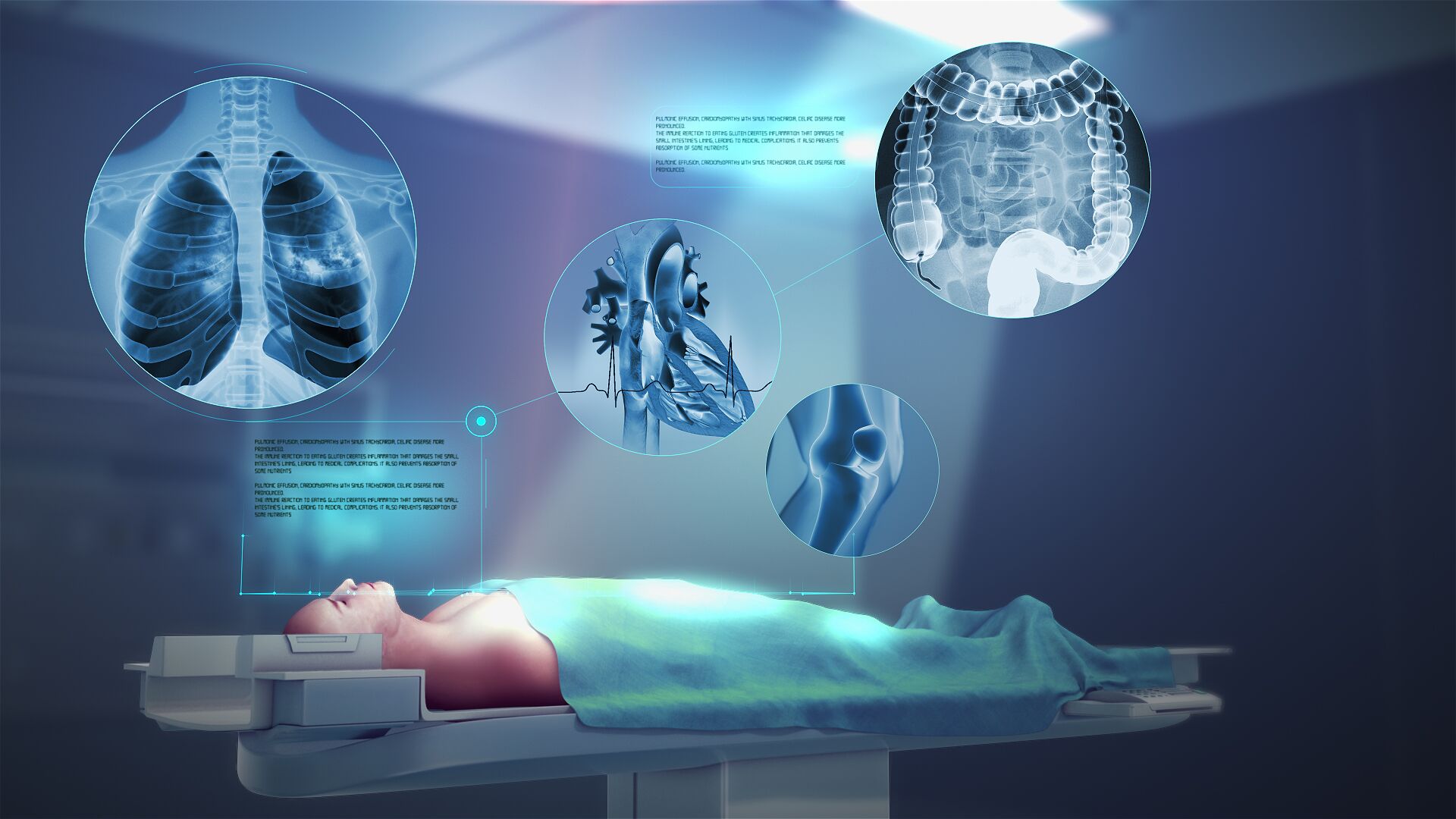
AI has long been touted as the next big thing in medicine. Now, a new study explains how analyzing complex and messy healthcare data is becoming easier because of machine learning.
As per a study published in the Nature Digital Medicine, it is now possible to predict for patients, their duration of hospital stay, sudden readmission, and even the probability of death, more accurately than with conventional predictive models. The algorithm has been used to check the health records of 216,000 adult patients so far.
This has implications for artificial intelligence (machine learning) in medicine:
How does Deep Learning in AI benefit Healthcare?
Deep learning uses multiple layers of non-linear processing units to understand data, classify it or make predictions. The study is an example of how deep learning applies to medical prediction tasks. The neural networks, unlike the traditional predictive models, can sift through piles of unorganized raw data and organize it through variables that are important in predicting health outcomes. In other words, not only does Deep Learning make predictions based on the most important variables, it also determines what the most important variables are in the first place.
As a result, the study demonstrates that meaningful, actionable predictions can be made by processing vast amounts of unstructured, messy data. These data, previously considered unusable, include clinical notes, labels or data with large numbers of variables, which may or may not be well-structured and may even contain errors.
New learnings from this predictive model
Predictive models are designed to help the machine predict patient’s health and, hence, assist the doctor to decide on the best follow-up action and also have an idea of how and when to act.
The algorithm enables machine learning to read a retinal image, detect patients who likely have a genetic condition that can lead to a fatal heart attack at a premature age, and improve outcomes through early diagnosis of disease.
Prime ethical concerns
Biased data may lead to unfair results: The algorithms designed are prone to accidentally institutionalize existing human biases, such as racial bias that might be present in the data.
Effect on the doctor-patient relationship: Confidentiality, which is the core value of healthcare, begins to weaken. A doctor visit gets replaced by a healthcare-system visit that has a care team and electronic records about you.
What next?
Stanford is actively working to bring AI to the clinic in the next few years. Overall, the immense scope of AI in the pharma industry is well understood. The judicious application of AI will govern the overall benefit to healthcare and to society.
Disclaimer: The above information has been sourced from Stanford Medicine blog. The information in no way constitutes, or should be construed as medical advice. Nor is the above article an endorsement of any research findings discussed in the article an endorsement for any of the source publications.